2024-08-21
【學術亮點-頂級會議論文】基於標籤依賴性和特徵相似性間動態關係的細粒度屬性預標記方法RGBT2HS-Net:利用注意力驅動多重解析度架構來從RGB-T影像重建高光譜影像堆疊
Font Size
Small
Middle
Large
【學術亮點-頂級會議論文】基於標籤依賴性和特徵相似性間動態關係的細粒度屬性預標記方法RGBT2HS-Net:利用注意力驅動多重解析度架構來從RGB-T影像重建高光譜影像堆疊
AI core Technology: Big Data Platform in Agriculture and Data Governance【Institute of Data Science and Information Computing / Hao-Chiang Shao / Assistant Professor】
核心技術:農業大數據共享服務平台及資料治理【資料科學與資訊計算研究所邵皓強助理教授】
上架日期2024-04-19
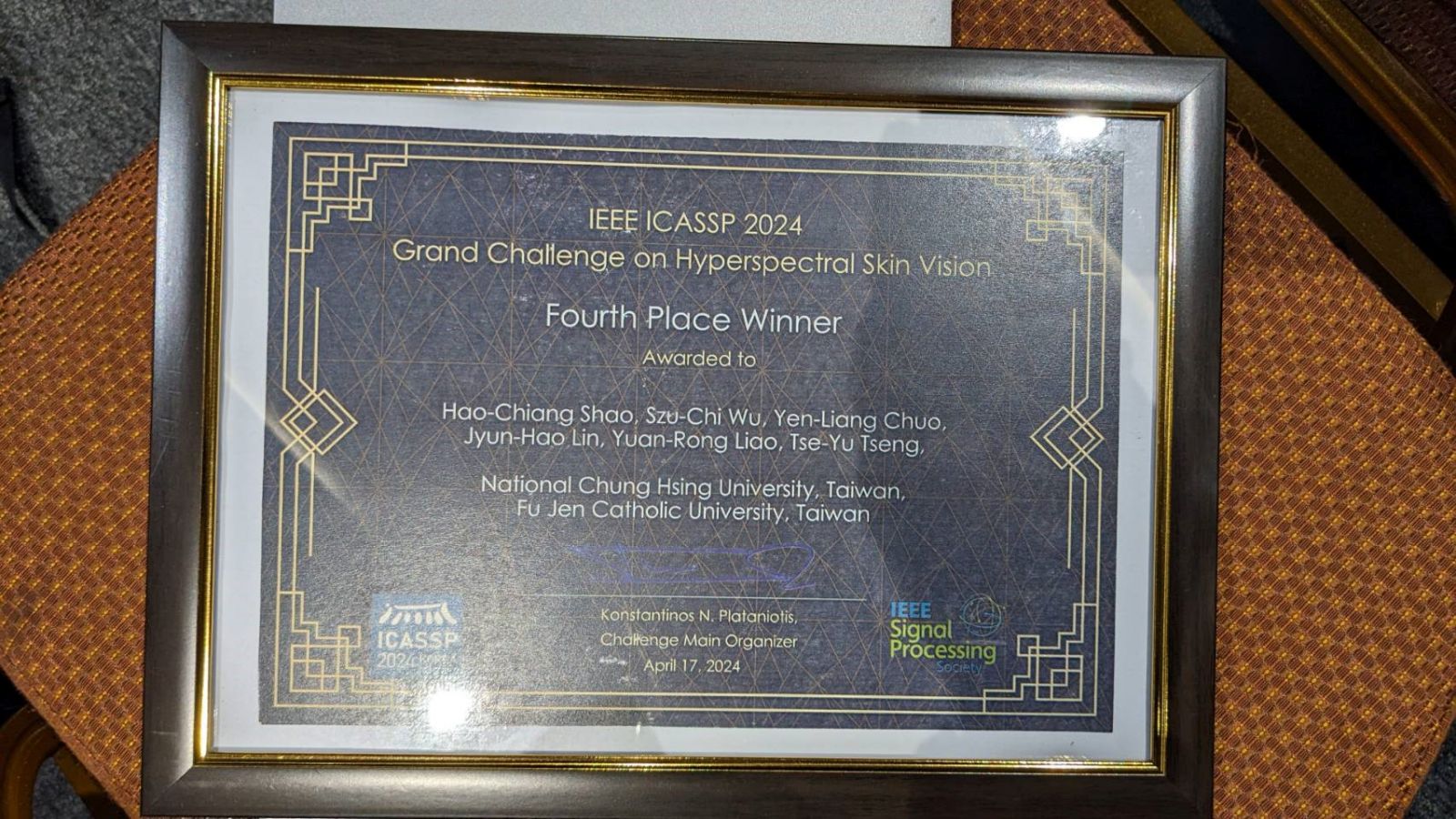
競賽第四名獎狀
AI core Technology: Big Data Platform in Agriculture and Data Governance【Institute of Data Science and Information Computing / Hao-Chiang Shao / Assistant Professor】
核心技術:農業大數據共享服務平台及資料治理【資料科學與資訊計算研究所邵皓強助理教授】
論文篇名 | 英文:RGBT2HS-Net: Reconstructing a Hyper-Spectral Volume from an Rgb-T Stack via an Attention-Powered Multiresolution Framework 中文:RGBT2HS-Net:利用注意力驅動多重解析度架構來從RGB-T影像重建高光譜影像堆疊 |
期刊名稱 | Proceedings of IEEE International Conference on Acoustics, Speech and Signal Processing 2024 (指標清單期刊) |
發表年份, 卷數, 起迄頁數 | 2024, pp.83-84 (ICASSP 2024, Grand Challenges 競賽論文) |
作者 | Hao-Chiang Shao(邵皓強)*, Szu-Chi Wu, Yen-Liang Chuo, Jyun-Hao Lin, Yuan-Rong Liao, Tse-Yu Tseng |
DOI | 10.1109/ICASSPW62465.2024.10627758 |
中文摘要 | 在此競賽論文中,我們提出了RGBT2HS-Net方法,該方法能夠從4個頻道的「RGB-熱成像堆疊」來重建可見光與近紅外光譜範圍內的61通道高光譜影像信號。RGBT2HS-Net具有兩個主要特點。首先,它採用多重解析度框架,允許透過可學習濾波器分解4D RGB-T影像為多重解析度張量,以利後續處理。其次,它結合了注意力機制和可擴展的感受域(receptive field),使得能夠學習頻道間依賴性(inter-channel dependency)和頻道內相關性(intra-channel correlation),以有效重建高光譜影像信號。實驗結果顯示,我們的方法在驗證資料集上能得到平均大於27 dB 的PSNR值,同時,在官方測試資料集上的SAM(spectral angle mapper)分數也優於既有最先進的模型。我們所提出的模型架構,具有優秀的高光譜影像訊號重建能力。 |
英文摘要 | We present our RGBT2HS-Net method, capable of reconstructing the 61-channel spectral reflectance in the visible and near-infrared spectral range from a 4-channel RGB-Thermal image stack. RGBT2HS-Net has two primary characteristics. First, it employs a multiresolution framework, allowing the input 4D RGB-Thermal image stack to be decomposed into multiresolution tensors via learnable filters for further processing. Second, it incorporates an attention mechanism with a scalable receptive field, enabling the learning of both inter-channel dependencies and intra-channel correlations for hyperspectral reconstruction. Experiments demonstrate that our method outperforms previous state-of-the-art models in terms of the SAM score on the testing set and achieves an average PSNR greater than 27 dB on the validation set. |
發表成果與AI計畫研究主題相關性 | 此項競賽的目標是從日常相機捕獲的 RGB 影像中重建可見光 (VIS) 和近紅外線 (NIR) 光譜範圍內的皮膚光譜反射率,為化妝品和美容應用提供變革性方法。 透過重建可見光和近紅外光譜中的皮膚光譜反射率,希望在較平價且一般人消費的起的設備上存取豐富的高光譜資訊。借助重建的皮膚光譜,為直接透過消費者的智慧型手機和其他設備創建個人化美容和護膚解決方案。 此競賽為本年度ICASSP Signal Processing Grand Challenge的第5號競賽,共有51支國際隊伍參賽,最後僅有9隊完成高光譜影像的重建任務,我們的RGBT2HS-Net則獲得國際第4名。此為信號重建之AI技術,故與計畫主題相關。 |
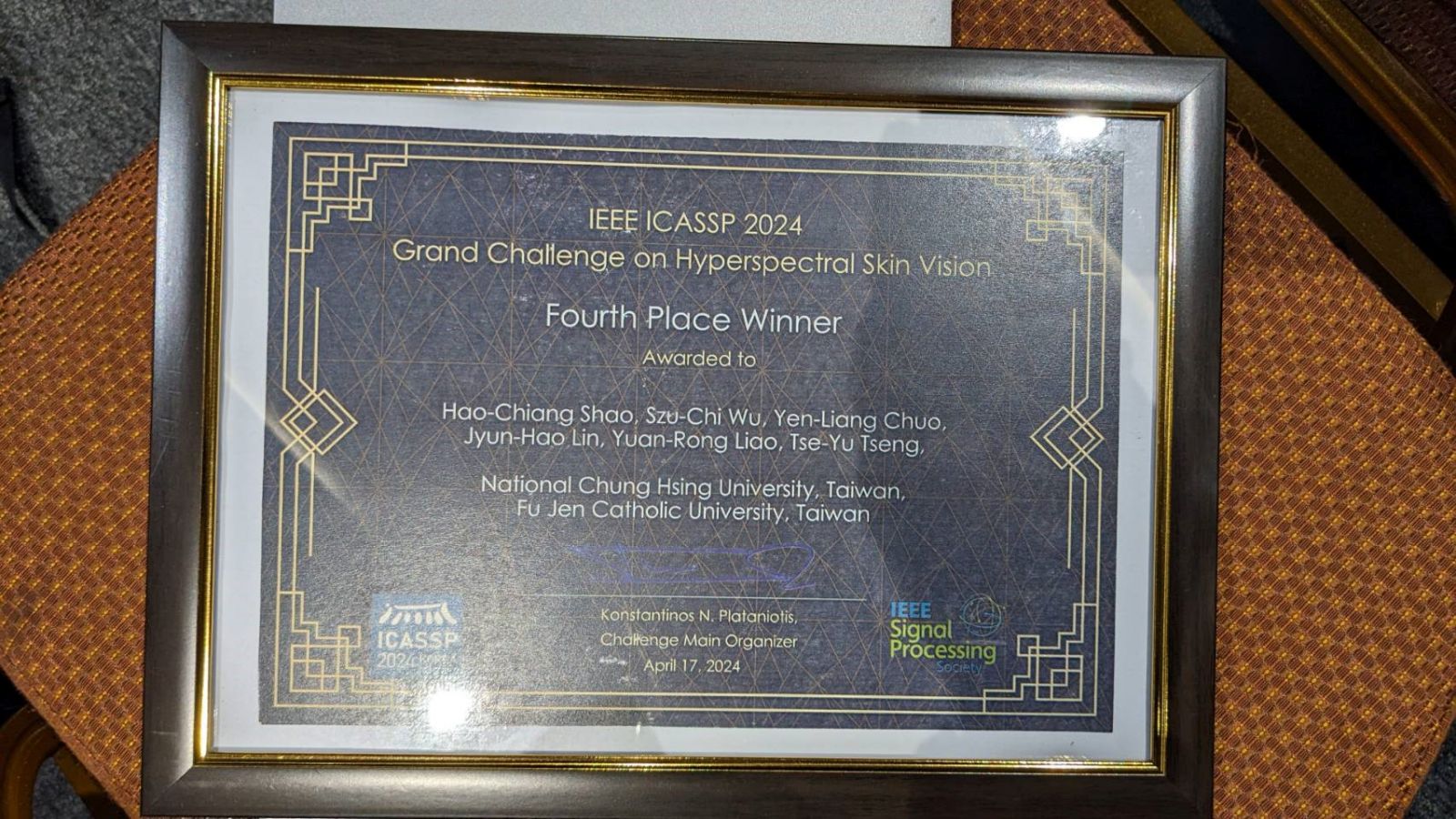
競賽第四名獎狀